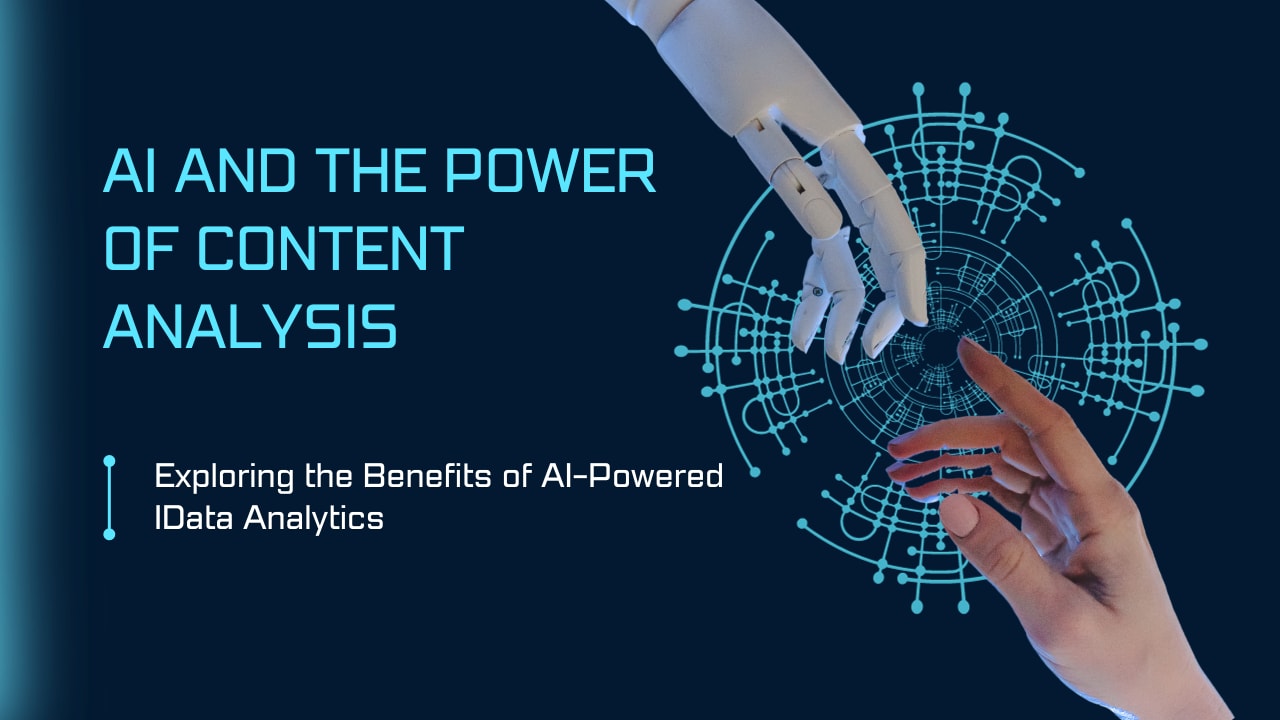
In the modern world, we see endless content on social media through images, text, and videos. With loads of content generated each second, it becomes challenging for businesses to analyze the trends, hot topics, and themes.
But AI has simplified the process. Today, enterprises and healthcare centers leverage intelligent algorithms to sort, clean, analyze, and extract meaning from raw data. It is fast and demands zero effort from humans. Using AI for content analysis helps businesses keep up with trends and improve customer service.
Want to know more about AI content analysis to help you make better decisions for your business? Let us proceed and check the role of deep learning and natural language processing in interpreting unstructured information.
In this article
Part I. Understanding AI Content Analysis
AI content analysis refers to the automated analysis of large content with the help of AI technologies. It can be defined as interpreting unstructured content using artificial intelligence. The content can be in multiple formats, including text, images, videos, and diagrams. AI content analytics use advanced algorithms and NLP to extract keywords, entities, and patterns from existing data.
AI-powered content analysis is widely used in competitor research, customer service, healthcare, and security. This technology helps businesses obtain accurate analysis, preventing operational errors. It also assists in improving productivity by reducing the manual workload. Here are some of the highlighting advantages of AI content analysis.
Advantages of AI Content Analysis
- Scaling data analysis is now quicker with AI. It trains the system to process loads of information without manual work.
- It can detect deeper correlations a human mind may never apprehend. Therefore, the outcome accuracy of AI analytics is incomparable.
- Modern NLP algorithms enable content analytics to read the sentiments and tone of data. This offers meaningful insight into what a customer feels about the product.
- AI analysis tools also help companies in content curation. Businesses can research hot topics and themes for a specific sample audience.
- Advanced AI video analysis tool helps you monitor activity in real time. It aids law enforcement agencies in making timely decisions.
Part II. Types of AI-powered Content Analysis
1. Text Analysis
Text analysis is a field of study that involves extracting meaningful insights and information from textual data. It encompasses a range of techniques and methods to analyze and understand the structure, content, and context of text documents.
For example, you could use AI to summarize the content of a passage. A typical use case would be the Smart Summary function of Edraw AI, a feature analysis of diagram maker EdrawMax, which leverages AI algorithms to summarize text. It can be especially helpful if you need to make a diagram like a flowchart, a mind map, or a timeline with large chunks of text to deal with.
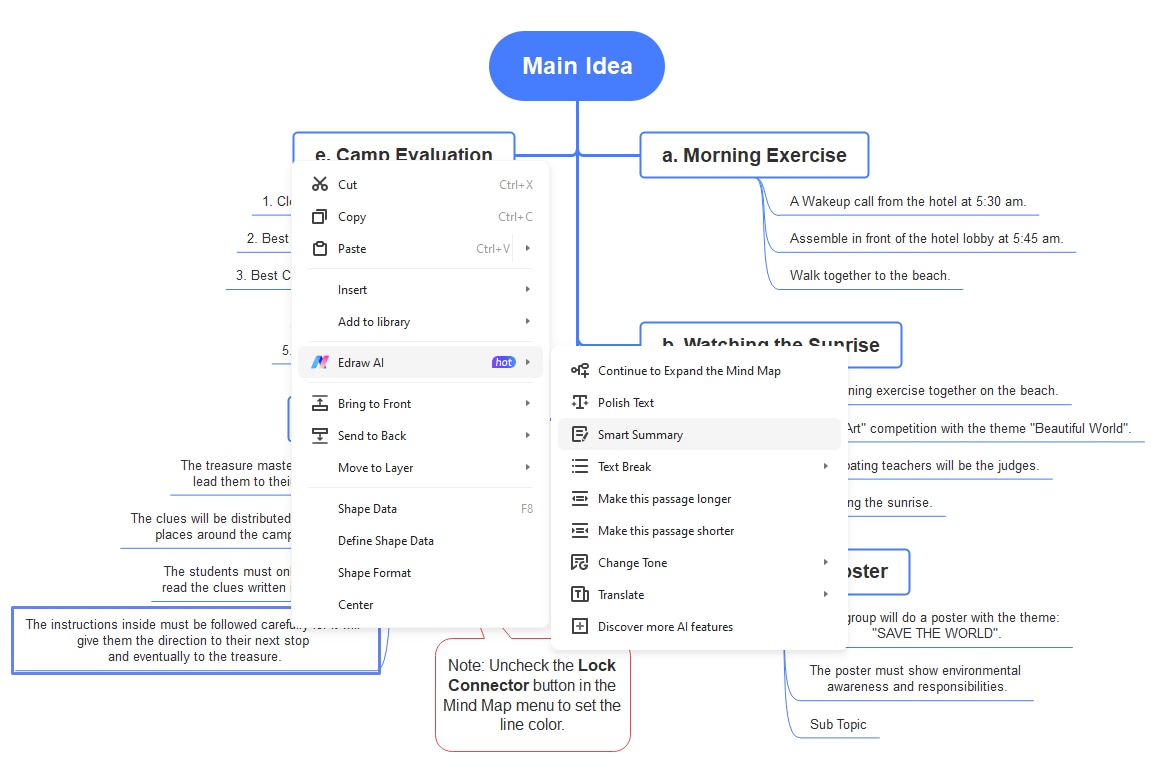
2. Diagram analysis
In addition to text, AI has also been used to analyze images. For example, AI has been widely applied to extract text from pictures.
OCR
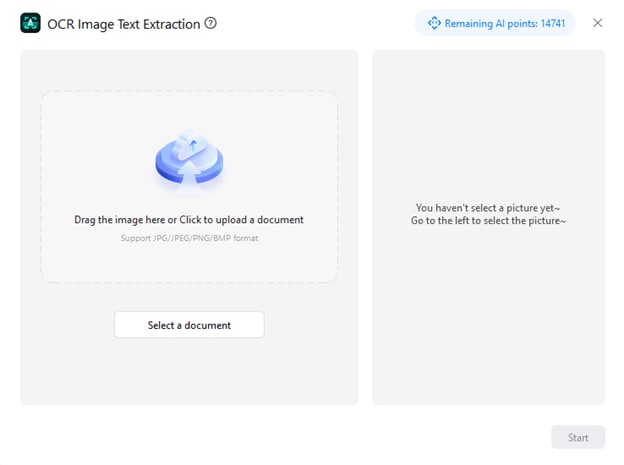
Chart Analysis
You can now also use AI to analyze common charts like bar charts, line graphs, flowcharts, and Gantt charts. AI can provide a description of your charts, detect errors, and make optimization suggestions.
For example, Edraw AI’s Flowchart Analysis function supports the detection of flowchart flaws, optimization, and description.
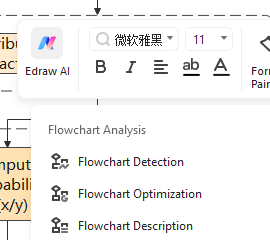
Its Gantt chart analysis function can generate a project overview, and project progress report, and identify risks to prevent bottlenecks during implementation.
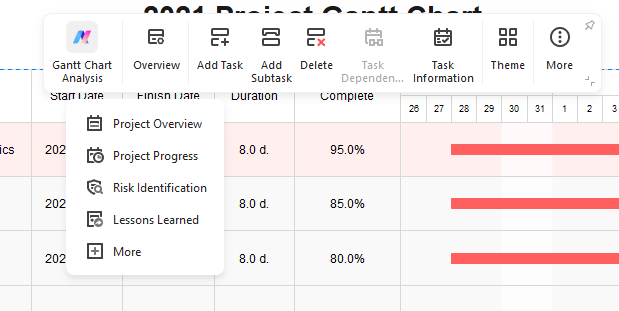
Its Chart Analysis function has to compare data, identify anomalies, summarize content, and predict trends of other kinds of charts.
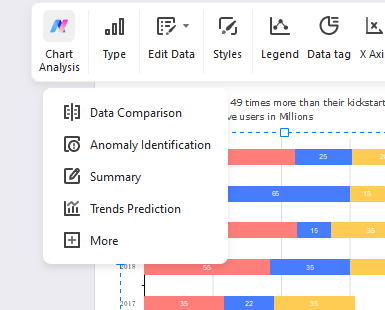
3. Video Analysis
AI Video analysis is analyzing human behavior in videos through machine learning. Modern AI video analytics use real-time videos and post-recordings to extract insights. Video analytics trains modern systems using DNNs or Deep Neural Networks. These networks combine objective detection and activity recognition to analyze the content.
First, the algorithm classifies and labels moving images. Next, it localizes the selected image and categorizes objects. Then the system triggers alerts once the object is identified by tracking a specific activity or event.
Video analysis is widely used in transport. It manages traffic jams and prevents accidents on busy roads. Hospitals also use AI video analysis to monitor abductions and drug diversions. The surveillance tools also aid in at-home monitoring of elderly and disabled patients. In addition, trainers post-process match videos to analyze players’ techniques and improve results.
4. Cross-Modal Analysis
Cross-modal learning is a technique that analyzes data from multiple modalities. It leverages intelligent, versatile algorithms to understand, evaluate, and interpret diverse information. Typically, this approach is used when one modality is incomplete and needs content to fill up spaces. Here are the two popular techniques used in cross-modal analysis.
- Real-Value Representation: It focuses on using different modalities for analyzing one event. This approach involves using deep learning to understand the shared topic and joint representation of a subject. For instance, a text describing “a picture with cherry blossom” will have a similar visual cue.
- Binary-Value Representation: In comparison, this approach involves using any data modalities in binary codes. Giving each modality a different hash/ binary character makes it easier to compare them.
Part III. Best Practices for Efficient AI Content Analysis
AI content analytics are only beneficial when used effectively. Here are some practices every business should consider before interpreting content using analytics.
Data Preparation and Cleaning
The output of content analytics relies highly on the quality of data. So, what’s better is to obtain first-party data that is identifiable and traceable. In the case of using third-party data, opt for a transparent source. Remove unnecessary clutter and organize the datasets.
Choosing the Right Tools
There is a wide array of content analysis tools available to extract meaning and interpret information. This is why teams should clearly understand the objective and key performance indicators. It will help narrow down the algorithms and software.
Evaluating and Iterating Analysis Results
AI content analytics are undoubtedly revolutionary in customer service, but they are not the all-in-one solution. You still need human intuition and collaboration to utilize them to your needs. So, it is best for businesses to make iterating strategies for better analysis procedures.
Part IV. Ethical Considerations in AI Content Analysis
Businesses should approach content analysis with due ethical considerations. It builds brand-customer trust and avoids negative consequences. Here are some ethical duties for any analysts using a large dataset for interpretations.
- The participants or customers should be informed about the handling of data. Businesses must educate them about the research purpose and methods beforehand.
- The datasets should be accurate and free from misinformation. It is understandable that there will be limitations in doing so. In this case, analysts must be vocal about these limitations.
- Analysts should be transparent about factors that influence their results. It can be unrepresented samples, biased data collection methods, and more.
- It is significant for data analysts to respect ownership rights. They should acknowledge the intellectual property rights of the sites from where the data is collected.
- Businesses should make sure their outcomes are not misused.
Part V. Future Trends and Developments in AI Content Analysis
Here are some notable advancements in AI content analysis.
Video Analysis
Video analysis and computer vision is undoubtedly the fastest-growing sector of data analytics. Modern data analytics are able to interpret activities in real time. Hence, businesses, healthcare centers, and security sectors are more than ready to take urgent action.
Automation
Intelligent automation has completely eliminated the need for lengthy tasks. Now, businesses can sort and clean massive datasets using machine automation tools. It streamlines the process and boosts productivity.
Enhanced Predictive Analytics
AI has come a long way with its advanced algorithms. Modern companies are now using these network algorithms to use historical and modern data for analysis. It makes businesses foresighted and creates new opportunities for innovation.
Conclusion
The emergence of AI in data analysis has revolutionized the industry. Over the years, developments in NLP and deep learning have made interpreting loads of unstructured information easy. Thus, businesses can now efficiently streamline their work and satisfy customers.
Moreover, modern businesses are well-equipped to make data-driven decisions. This is primarily accredited to intelligent tools like EdrawMax. They derive feedback from raw text and lengthy diagrams with a few clicks. If your enterprise needs help visualizing the feedback output, you might want to try it out.
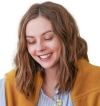